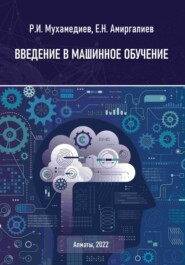
Полная версия:
Введение в машинное обучение
35
Дьяконов А. Г. Анализ данных, обучение по прецедентам, логические игры, системы WEKA, RapidMiner и MatLab (Практикум на ЭВМ кафедры математических методов прогнозирования): учебное пособие. – М.: Изд. отдел факультета ВМК МГУ им. М. В. Ломоносова, 2010.
36
Martin Fodslette Møller. A scaled conjugate gradient algorithm for fast supervised learning // Neural Networks. – 1993. – Vol. 6. – Issue 4. – P. 525–533.
37
Dong C. Liu, Jorge Nocedal. On the limited memory BFGS method for large scale optimization // Mathematical Programming. – 1989. – Vol. 45. – Issue 1–3. – P. 503–528.
38
Derivative of Cost Function for Logistic Regression. – https://medium.com/mathematics-behind-optimization-of-cost-function/derivative-of-log-loss-function-for-logistic-regression-9b832f025c2d
39
Warren S. McCulloch, Walter Pitts. A logical calculus of the ideas immanent in nervous activity // The bulletin of mathematical biophysics. – 1943. – Vol. 5. – Issue 4. – P. 115–133.
40
Rosenblatt, F. The perceptron: A probabilistic model for information storage and organization in the brain // Psychological Review. – 1958. – Vol. 65 (6). – P. 386–408.
41
Minsky M. L., Papert S. A. Perceptrons: An Introduction to Computational Geometry. – MIT, 1969. – 252 p.
42
Marvin Minsky, Seymour Papert. Perceptrons, expanded edition. – The MIT Press, 1987. – 308 p.
43
Werbos P. Beyond Regression: New Tools for Prediction and Analysis in the Behavioral Sciences. – Harvard University, 1974. – 38 p.
44
Werbos P. J. Backpropagation: past and future // IEEE International Conference on Neural Networks. – San Diego, 1988. – Vol. 1. – P. 343–353.
45
Нейрокомпьютеры: учеб. пособие для вузов. – М.: Изд-во МГТУ им. Н. Э. Баумана, 2004. – 320 с.
46
Галушкин А. И. Решение задач в нейросетевом логическом базисе // Нейрокомпьютеры: разработка, применение. – М.: Радиотехника, 2006. – № 2. – С. 49–71.
47
Ясницкий Л. Н. Введение в искусственный интеллект: учебное пособие для вузов. – М.: Академия, 2008. – 176 с.
48
Галушкин А. И. Нейронные сети: основы теории. – Горячая линия – Телеком, 2010. – 496 с.
49
Connectionism. Internet Encyclopedia of Philosophy. –https://iep.utm.edu/connect/#:~:text=Connectionism%20is%20an%20approach%20to,%2C%20neuron%2Dlike%20processing%20units
50
David Saad. Introduction. On-Line Learning in Neural Networks. – Cambridge University Press, 1998. – P. 3–8.
51
Cybenco G. Approximation by superpositions of a sigmoidal function // Mathematics of Control, Signals, and Systems. – 1989. – Vol. 4. – P. 304–314.
52
Hornik K. et al. Multilayer feedforward networks are universal approximators // Neural Networks. – 1989. – Vol. 2. – P. 359–366.
53
Schmidhuber, Jürgen. Deep learning in neural networks: An overview // Neural Networks. – 2015. – Vol. 61. – P. 85–117.
54
http://www.asimovinstitute.org/neural-network-zoo/ – THE NEURAL NETWORK ZOO POSTED ON SEPTEMBER 14, 2016 BY FJODOR VAN VEEN
55
Werbos P. Beyond Regression: New Tools for Prediction and Analysis in the Behavioral Sciences. – Harvard University, 1974. – 38 p.
56
Batch, Mini-Batch & Stochastic Gradient Descent. – https://towardsdatascience.com/batch-mini-batch-stochastic-gradient-descent-7a62ecba642a
57
Обучи свою первую нейросеть: простая классификация. – https://www.tensorflow.org/tutorials/keras/classification
58
Dudani, Sahibsingh A. The Distance-Weighted k-Nearest-Neighbor Rule // Systems, Man, and Cybernetics. – 1976. – Vol. SMC-6. – Issue 4. – P. 325–327.
59
K-Nearest Neighbors algorithm. – http://en.wikipedia.org/wiki/K-nearest_neighbors_algorithm (2012-07-05).
60
Support vector machine. – http://en.wikipedia.org/wiki/Support_vector_machine (2012-02-22).
61
Classifier comparison. – https://scikit-learn.org/stable/auto_examples/classification/plot_classifier_comparison.html
62
Коллаборативная_фильтрация. – ru.wikipedia.org/wiki/Коллаборативная_фильтрация; https://en.wikipedia.org/wiki/Collaborative_search_engine
63
Friedman, Jerome H. Greedy function approximation: a gradient boosting machine // Annals of Statistics. – 2001. – P. 1189–1232.
64
Бустинг. – http://www.machinelearning.ru/wiki/index.php?title=Бустинг
65
Pearson K. On lines and planes of closest fit to systems of points in space // Philosophical Magazine. – 1901. – Vol. 2. – P. 559–572.
66
Sylvester J. J. On the reduction of a bilinear quantic of the nth order to the form of a sum of n products by a double orthogonal substitution // Messenger of Mathematics. – 1889. – Vol. 19. – P. 42–46.
Вы ознакомились с фрагментом книги.
Для бесплатного чтения открыта только часть текста.
Приобретайте полный текст книги у нашего партнера:
Полная версия книги
Всего 10 форматов