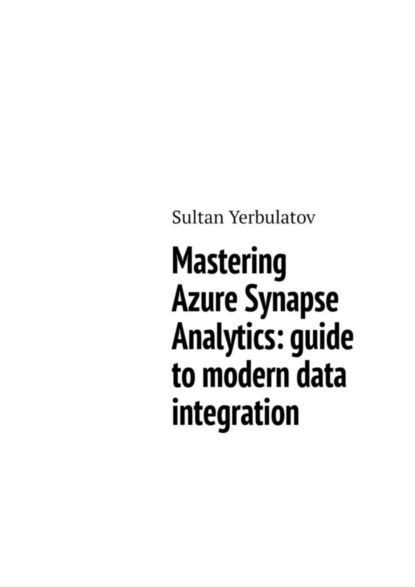
Полная версия:
Mastering Azure Synapse Analytics: guide to modern data integration

Mastering Azure Synapse Analytics: guide to modern data integration
Sultan Yerbulatov
© Sultan Yerbulatov, 2024
ISBN 978-5-0064-1399-3
Создано в интеллектуальной издательской системе Ridero
Welcome to «Mastering Azure Synapse Analytics: Guide to Modern Data Integration.» In this book, we embark on a journey through the intricate world of Azure Synapse Analytics, Microsoft’s cutting-edge cloud analytics service designed to empower organizations with powerful data integration, management, and analysis capabilities. Whether you’re a seasoned data professional looking to expand your skills or a newcomer eager to harness the full potential of Azure Synapse Analytics, this book is your comprehensive companion. Through detailed explanations, practical examples, and expert insights, we delve into the core concepts, best practices, and advanced techniques necessary to navigate the complexities of modern data analytics. From data ingestion and transformation to dynamic data masking, compliance reporting, and beyond, each chapter is meticulously crafted to provide you with the knowledge and skills needed to succeed in today’s data-driven world.
Throughout my career as a data engineer, I have had extensive hands-on experience with various data platforms, culminating in a deep expertise in Azure Synapse Analytics. This book draws on my practical knowledge and industry insights, providing readers with step-by-step instructions, best practices, and detailed examples of how to implement, optimize, and secure data solutions using Synapse Analytics. Key topics include data ingestion, integration with Power BI for reporting, ensuring compliance with data regulations, dynamic data masking, and advanced monitoring and troubleshooting techniques.
This book offers a thorough exploration of Azure Synapse Analytics, Microsoft’s powerful cloud analytics service that unifies big data and data warehousing. With a focus on real-world applications and technical depth, this book is designed to be an invaluable resource for data professionals, engineers, and business analysts who aim to leverage the full potential of Azure Synapse Analytics in their organizations.
I believe that «Mastering Azure Synapse Analytics» will meet the growing demand for comprehensive, authoritative resources on modern data analytics platforms. The book’s structured approach, combined with its practical focus, makes it suitable for both beginners and seasoned professionals seeking to deepen their understanding and enhance their skills.
Acknowledgments
I would like to express my sincere gratitude to all those who contributed to the creation of this book. Special thanks to my Data Engineering Chapter Architects in Tengizchevroil, namely Salimzhan Isspayev and Talgat Kuzhabergenov, whose invaluable insights and feedback helped shape the content and ensure its relevance and accuracy. I am also grateful to my other colleagues and mentors for their support and encouragement throughout this journey. Additionally, I extend my appreciation to the team at Data & Insights team for their professionalism and dedication in bringing this book to fruition. Lastly, I owe a debt of gratitude to my family and specifically my loved wife for their unwavering support and understanding during the writing process. This book would not have been possible without their encouragement and belief in my vision.
Chapter 1. Introduction
In today’s rapidly evolving digital landscape, businesses are generating vast amounts of data, creating an unprecedented demand for efficient data management, processing, and analytics tools. Azure Synapse Analytics, Microsoft’s’ all-in-one data solution, is here to revolutionize the world of data, providing a comprehensive platform for data storage, processing, visualization, machine learning, and more.
Understanding the Data Engineering Landscape
In an era where data is often hailed as the new oil, the role of data engineering in transforming raw information into valuable insights has become increasingly vital. Let’s embark on a journey through the intricate terrain of the data engineering landscape, exploring its key components, challenges, and the profound impact it has on diverse industries.
Data engineering serves as the backbone of modern analytics, acting as the bridge between data collection and meaningful interpretation. It encompasses a spectrum of activities, from designing robust data architectures to implementing efficient processing pipelines. To appreciate its significance, one must first grasp the evolution of data engineering over time.
From Silos to Integration
Traditionally, data was stored in isolated silos, making collaboration and analysis challenging. The advent of data engineering brought about a paradigm shift, encouraging the integration of diverse data sources into unified systems. Today, data lakes and warehouses stand as testaments to the power of consolidating information for comprehensive insights.
A fundamental aspect of understanding data engineering lies in recognizing its ecosystem. This ecosystem comprises key components, each playing a unique role in the data processing journey.
Data Storage Systems
From the vast expanses of data lakes to the structured warehouses meticulously organized for analytics, the variety of storage systems available reflects the diverse nature of data. NoSQL databases, with their flexibility, have become instrumental in handling unstructured data, providing a dynamic foundation for the modern data engineer.
Data Processing Technologies
Batch processing, where data is collected, processed, and stored in intervals, contrasts with the real-time allure of stream processing. Apache Hadoop and Spark are at the forefront, illustrating the engine power that fuels the processing capabilities of data engineering.
Data Integration Tools
The orchestration of data flows demands sophisticated tools. Platforms such as Apache NiFi and Azure Data Factory streamline the movement of data, ensuring a seamless journey from source to destination.
Data Quality: The Pillar of Reliability
In the realm of data engineering, the quality of data is paramount. Challenges such as inconsistent data, duplications, and missing elements are hurdles that must be addressed. Robust data quality frameworks and methodologies emerge as indispensable tools, safeguarding the integrity of the information that fuels decision-making processes.
Contemporary Practices and Trends
As technology advances, so do the practices within data engineering. Real-time data processing has shifted from being an aspiration to a necessity, enabling businesses to make informed decisions on the fly. Serverless architectures and the integration of artificial intelligence and machine learning further elevate the capabilities of data engineering, pushing the boundaries of what was once deemed possible.
A Glimpse into Real-world Applications
Concrete examples breathe life into the theoretical constructs of data engineering. Industries such as retail, healthcare, and finance leverage data engineering to enhance their operations. From optimizing inventory management in retail to predicting patient outcomes in healthcare, the impact of data engineering is ubiquitous.
Understanding the data engineering landscape opens a gateway to a dynamic world of opportunities. As we navigate through the complexities of storage, processing, and integration, we realize that the true power lies in transforming data into actionable insights. With each technological advancement, the landscape evolves, promising new horizons for data engineers ready to explore and innovate.
So, fasten your seatbelts and get ready to traverse the ever-expanding landscape of data engineering – a journey that promises not just data processing, but a transformation of how we perceive and utilize information.
1.2 Overview of Azure Synapse Analytics and the Key Components
Evolution of Azure Synapse Analytics: A Brief History
To understand the full significance of Azure Synapse Analytics, it’s essential to delve into its evolution. The story begins with the introduction of SQL Data Warehouse (SQL DW) by Microsoft. Launched in 2016, SQL DW was a remarkable product that aimed to combine the worlds of data warehousing and big data analytics. It was the first step towards creating an integrated platform for data storage and processing.
Over the years, as data grew in volume and complexity, the need for a more comprehensive solution became evident. In 2019, Microsoft rebranded SQL DW as Azure Synapse Analytics, marking a pivotal moment in the platform’s history. This rebranding represented a shift from just data warehousing to a more holistic data analytics service, encompassing data storage, processing, and advanced analytics.
With the rebranding came significant architectural changes and new features. Azure Synapse Analytics incorporated on-demand query processing, enabling users to perform ad-hoc queries without provisioning resources. This flexibility made it easier for organizations to adapt to fluctuating workloads and only pay for the resources they used.
The integration of Apache Spark, a powerful open-source analytics engine, further extended Azure Synapse Analytics’ capabilities. It allowed data engineers and data scientists to work with big data and perform advanced analytics within the same platform, simplifying the process of extracting valuable insights from data.
Azure Synapse Studio, introduced in 2020, became the central hub for data professionals to collaborate and manage their data workflows. It provided an integrated development environment that streamlined data preparation, exploration, and visualization, making it easier for teams to work together and derive meaningful insights.
Throughout its evolution, Azure Synapse Analytics maintained a strong focus on security and compliance, addressing the growing concerns surrounding data protection and governance. The platform continued to expand its list of certifications and compliance offerings to meet the stringent requirements of various industries.
In 2021, Azure Synapse Analytics introduced the Synapse Pathway program, designed to help businesses migrate from their existing data warehouses to the platform seamlessly. This program included tools and resources to facilitate a smooth transition and maximize the value of Azure Synapse Analytics.
Today, Azure Synapse Analytics stands as a testament to Microsoft’s commitment to providing a comprehensive data analytics solution. Its evolution from SQL Data Warehouse to a holistic data platform has made it a go-to choice for organizations looking to harness the power of their data. As technology and data continue to advance, Azure Synapse Analytics is sure to adapt and evolve, keeping businesses at the forefront of data-driven innovation.
In this chapter, we delve into the many facets of Azure Synapse Analytics to understand how it can reshape the way we interact with data.
Data Storage:
Azure Synapse Analytics offers robust data storage capabilities that are crucial for its role as a data warehousing solution. It combines both data warehousing and Big Data analytics to provide a comprehensive platform for storing and managing data. Here are more details about data storage in Azure Synapse Analytics:
– Distributed Data Storage: Azure Synapse Analytics leverages a distributed architecture to store data. It uses a Massively Parallel Processing (MPP) system, which divides and distributes data across multiple storage units. This approach enhances data processing performance by enabling parallel operations.
– Data Lake Integration: Azure Synapse Analytics seamlessly integrates with Azure Data Lake Storage, a scalable and secure data lake solution. This integration allows organizations to store structured, semi-structured, and unstructured data in a central repository, making it easier to manage and analyze diverse data types.
– Columnstore Indexes: Azure Synapse Analytics uses columnstore indexes, a storage technology optimized for analytical workloads. Unlike traditional row-based databases, columnstore indexes store data in a columnar format, which significantly improves query performance for analytics and reporting.
– Polybase: Azure Synapse Analytics includes Polybase, which enables users to query data across different data sources, such as relational databases, data lakes, and external sources like Azure Blob Storage and Hadoop Distributed File System (HDFS). This feature simplifies data access and analysis by centralizing data sources.
– Data Compression: The platform employs data compression techniques to optimize storage efficiency. Compressed data requires less storage space and improves query performance. This is particularly beneficial when dealing with large datasets.
– Data Partitioning: Azure Synapse Analytics allows users to partition data tables based on specific criteria, such as date or region. Partitioning enhances query performance because it limits the amount of data that needs to be scanned during retrieval.
– Security and Encryption: Data security is a top priority in Azure Synapse Analytics. It offers robust security features, including data encryption at rest and in transit. Users can also implement role-based access control (RBAC) model and integrate with Azure Active Directory to ensure that only authorized users can access and manipulate the data.
– Data Distribution: The platform allows users to specify how data is distributed across nodes in a data warehouse. Proper data distribution is crucial for query performance. Azure Synapse Analytics provides options for distributing data through methods like round-robin, hash, or replication, based on the organization’s specific needs.
– Data Format Support: Azure Synapse Analytics supports various data formats, including Parquet, Avro, ORC, and JSON. This flexibility enables organizations to work with data in the format that best suits their analytics needs.
Data Processing
When it comes to data processing, Azure Synapse Analytics truly shines. It combines on-demand and provisioned resources for massive parallel processing, allowing organizations to handle large volumes of data quickly and efficiently. The seamless integration of Apache Spark and SQL engines makes data processing a breeze. By combining these powerful engines, organizations can leverage the strengths of both worlds – SQL for structured data and analytics, and Apache Spark for big data processing and machine learning. Here’s a more detailed look at this integration:
Apache Spark Integration benefits: Unified Data Processing. Azure Synapse Analytics supports the integration of Apache Spark, an open-source, distributed computing framework. This allows users to process and analyze both structured and unstructured data using a single platform.
Big Data Processing: Apache Spark is known for its capabilities in handling big data. With this integration, organizations can efficiently process large datasets, including those stored in Azure Data Lake Storage or other data sources.
Machine Learning: Spark’s machine learning libraries can be utilized within Azure Synapse Analytics. This enables data scientists and analysts to develop and deploy machine learning models using Spark’s capabilities, helping organizations gain valuable insights from their data.
SQL Engine Integration benefits: T-SQL Compatibility. Azure Synapse Analytics uses T-SQL (Transact-SQL) as the query language, providing compatibility with traditional SQL databases. This makes it easier for users with SQL skills to transition to the platform.
Data Warehousing: The SQL engine within Synapse Analytics is optimized for data warehousing workloads, making it an ideal choice for structured data analysis and reporting.
Advanced Analytics: Users can run advanced analytics queries and functions using T-SQL. This includes window functions, aggregations, and complex joins, making it suitable for a wide range of analytics scenarios.
In-Database Analytics: The SQL engine supports in-database analytics, allowing users to run complex analytics functions within the data warehouse. This minimizes data movement and accelerates analytics.
Data Visualization
Data without insights is just raw information. Azure Synapse Analytics seamlessly integrates with Microsoft Power BI, a powerful data visualization and business intelligence tool. Users can create visually appealing and interactive reports and dashboards by connecting Power BI to their Azure Synapse Analytics data. This integration allows for real-time data exploration and visualization. It’s a game-changer for data-driven decision-making.
Machine Learning
Azure Machine Learning was a separate service, but it was possible to integrate it with Azure Synapse Analytics to enable machine learning capabilities within Synapse Analytics workflows. Since technology and services evolve rapidly, please verify the current state of integration and features.
Here’s an overview of how Azure Machine Learning can be used within Azure Synapse Analytics:
– Integration: Azure Machine Learning can be integrated into Azure Synapse Analytics to leverage the power of machine learning models in your analytics and data processing workflows. This integration allows you to access machine learning capabilities directly within Synapse Studio, the unified workspace for Synapse Analytics.
– Data Preparation: Within Synapse Studio, you can prepare your data by using data wrangling, transformation, and feature engineering tools. This is crucial as high-quality data is essential for training and deploying machine learning models.
– Model Training: Azure Machine Learning within Synapse Analytics lets you create and train machine learning models using a variety of algorithms and frameworks. You can select and configure the machine learning model that best suits your use case and data. Training can be done on a variety of data sources, including data stored in data lakes, data warehouses, and streaming data.
– Model Deployment: Once you’ve trained your machine learning models, you can deploy them within Synapse Analytics. These models can be used to make predictions on new data, allowing you to operationalize your machine learning solutions.
– Automated Machine Learning (AutoML): Azure Machine Learning offers AutoML capabilities, which can be used to automate the process of selecting the best machine learning model and hyperparameters. You can use AutoML to streamline the model-building process and find the best-performing model for your data.
Integration with Azure Services:
Azure Synapse Analytics seamlessly integrates with other Azure services, such as Azure Data Factory, Azure Machine Learning, and Power BI. This integration allows organizations to build end-to-end data solutions that encompass data storage, transformation, analysis, and visualization.
Pricing
Azure Synapse Analytics offers flexible pricing options, including on-demand and provisioned resources, allowing businesses to pay only for what they use. This flexibility, combined with its cost-management tools, ensures that you can optimize your data operations without breaking the bank.
Chapter 2. Getting Started with Azure Synapse Analytics
Embarking on the journey with Azure Synapse Analytics marks the initiation into a realm of unified analytics and seamless data processing. This comprehensive analytics service from Microsoft Azure is designed to integrate big data and data warehousing, providing a singular platform for diverse data needs. Whether you are a seasoned data engineer or a newcomer to the field, understanding the essential steps to get started with Azure Synapse Analytics is the key to unlocking its potential.
The journey into Azure Synapse Analytics is a dynamic exploration of tools and capabilities, each contributing to the seamless flow of data within the environment. In the subsequent chapters, we will continue to build upon this foundation, delving into advanced analytics with Apache Spark, data orchestration and monitoring, integration with Power BI for reporting, and the critical aspects of security, compliance, and cost management. As users become adept at navigating the intricacies of Azure Synapse Analytics, they unlock a world of possibilities for data engineering and analytics in the cloud.
2.1 Setting Up Your Azure Synapse Analytics Workspace
The first step in harnessing the capabilities of Azure Synapse Analytics is to set up your workspace. Navigating the Azure Portal, users can create a new Synapse Analytics workspace, defining crucial parameters such as resource allocation, geographic region, and advanced settings. This initial configuration lays the foundation for a tailored environment that aligns with specific organizational needs. As we dive into the setup process, we’ll explore how the choices made at this stage can significantly impact the efficiency and performance of subsequent data engineering tasks.
Setting up an Azure Synapse Analytics workspace is the first crucial step in leveraging the power of unified analytics and data processing. In this detailed guide, we’ll walk through the process, covering everything from creating the workspace to configuring essential settings.
Step 1: Navigate to the Azure Portal
– Open your web browser and navigate to the Azure Portal.
Step 2: Create a New Synapse Analytics Workspace
– Click on the “+«Create a resource» button on the left-hand side of the Azure Portal.
– In the «Search the Marketplace» bar, type «Azure Synapse Analytics» and select it from the list.
– Click the «Create» button to initiate the workspace creation process.
Step 3: Configure Basic Settings
– In the «Basic» tab, enter the required information:
– Workspace Name: Choose a unique name for your workspace.
– Subscription: Select your Azure subscription.
– Resource Group: Either create a new resource group or select an existing one.
Step 4: Advanced Settings
– Move to the «Advanced» tab to configure additional settings:
– Data Lake Storage Gen2: Choose whether to enable or disable this feature based on your requirements.
– Virtual Network: Configure virtual network settings if necessary.
– Firewall and Virtual Network: Set up firewall rules and virtual network rules to control access to the workspace.
Step 5: Review + Create
– Click on the «Review + create» tab to review your configuration settings.
– Click the «Create» button to start the deployment of your Synapse Analytics workspace.
Step 6: Deployment
– The deployment process may take a few minutes. You can monitor the progress on the Azure Portal.
– Once the deployment is complete, click on the «Go to resource» button to access your newly created Synapse Analytics workspace.
Step 7: Accessing Synapse Studio
– Within your Synapse Analytics workspace, navigate to the «Overview» section.
– Click on the «Open Synapse Studio» link to access Synapse Studio, the central hub for data engineering, analytics, and development.
Step 9: Integration with Azure Active Directory (Optional)
– For enhanced security and user management, integrate your Synapse Analytics workspace with Azure Active Directory (AAD). This can be done by navigating to the «Security + networking» section within the Synapse Analytics workspace.